LASSO when conditioning on less: ROSI¶
Instead of conditioning on the active set and signs, one can work in the full model and for each feature \(j\) selected construct p-values and confidence intervals conditional only on the event \(j\) was selected. This is the approach of Liu et al., which can be extended as ROSI (Relevant One-step Selective Inference) beyond squared-error loss (described in forthcoming work, though code is already available).
[1]:
import numpy as np, pandas as pd
import matplotlib.pyplot as plt
import statsmodels.api as sm
%matplotlib inline
from selectinf.tests.instance import gaussian_instance # to generate the data
from selectinf.algorithms.api import ROSI
We will know generate some data from an OLS regression model and fit the LASSO with a fixed value of \(\lambda\). In the simulation world, we know the true parameters, hence we can then return pivots for each variable selected by the LASSO. These pivots should look (marginally) like a draw from np.random.sample
. This is the plot below.
[2]:
np.random.seed(0) # for replicability
def simulate(n=500,
p=100,
s=5,
signal=(5, 10),
sigma=1):
# description of statistical problem
X, y, truth = gaussian_instance(n=n,
p=p,
s=s,
equicorrelated=False,
rho=0.,
sigma=sigma,
signal=signal,
random_signs=True,
scale=False)[:3]
sigma_hat = np.linalg.norm(y - X.dot(np.linalg.pinv(X).dot(y))) / np.sqrt(n - p)
L = ROSI.gaussian(X, y, 2 * np.sqrt(n), sigma=sigma_hat)
soln = L.fit()
active_vars = soln != 0
if active_vars.sum() > 0:
projected_truth = np.linalg.pinv(X[:, active_vars]).dot(X.dot(truth))
S = L.summary(truth=projected_truth)
S0 = L.summary()
pivot = S['pval'] # these should be pivotal
pvalue = S0['pval']
return pd.DataFrame({'pivot':pivot,
'pvalue':pvalue})
Let’s take a look at what we get as a return value:
[3]:
while True:
df = simulate()
if df is not None:
break
df.columns
[3]:
Index(['pivot', 'pvalue'], dtype='object')
[4]:
dfs = []
for i in range(200):
df = simulate()
if df is not None:
dfs.append(df)
[5]:
results = pd.concat(dfs)
import statsmodels.api as sm
grid = np.linspace(0, 1, 101)
fig = plt.figure(figsize=(8, 8))
plt.plot(grid, sm.distributions.ECDF(results['pivot'])(grid), 'b-', linewidth=3, label='Pivot')
plt.plot(grid, sm.distributions.ECDF(results['pvalue'])(grid), 'r-', linewidth=3, label='P-value')
plt.plot([0, 1], [0, 1], 'k--')
plt.legend(fontsize=15);
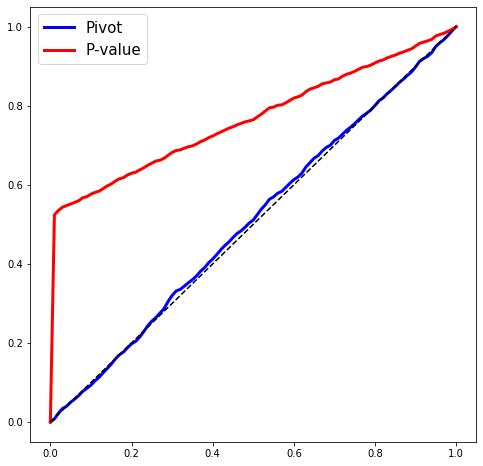